By definition, innovation requires breakthrough thinking and the corresponding development of new solutions. But what informs that thinking? Machine learning and data science are powerful tools that can speed the innovation process and yield better results, faster.
In today’s era of sophisticated data capture, behavior tracking, connected devices, social platforms and sensor-enabled products, data represents a new “natural resource” that nearly every company already possesses in one form or another. The question is this: How can organizations leverage this valuable data resource in order to support and amplify a culture of innovation?
The key is a smart approach rooted in data science. Applied data science entails more than data collection and basic analysis; it is sophisticated and responsive, able to iterate to produce optimal results. True data science requires not only knowledge of how to build machine learning and advanced analytic models, but the skill to understand business strategy — and the ability to link it all together.
First, ask the right questions in the right way
A data science professional must be able to communicate effectively across all areas of the organization, take business questions and translate them into a workable data science solution. This requires experience in assessing the types of data available, so that a machine learning solution can be developed to answer key questions posed by the business. Those answers can then be used as input for a cycle of innovation.
These questions are likely to be simple, but finding the answers may not be: this is why data science and machine learning are so powerful. For the marketing function the key questions may be: “Which campaigns have been the most successful? Why, and how can we predict which ones will be successful in the future?” For operations it may be: “Which products are likely to have warranty issues? Why, and how can we predict these issues ahead of time and mitigate the problem?”
Answering these types of business questions with machine learning and a data science methodology leads to game-changing insights that go beyond conventional data analytics and reporting. The result is true competitive advantage based on innovative deployment of new, data-driven models.
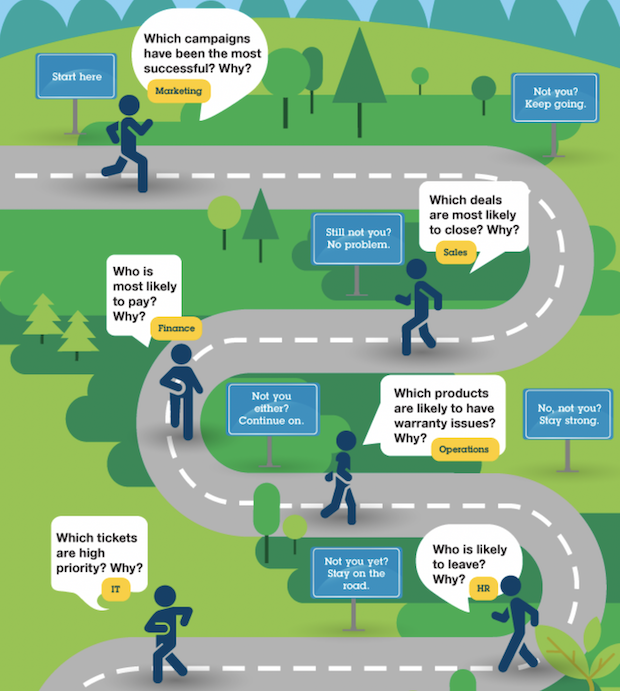
Next, build data science into the innovation cycle
With any new product or service design and development, part of the innovation process must involve understanding how data can be leveraged as a key innovation component. Smart organizations understand how to embed machine learning and AI into the concept at the beginning of development, in conjunction with the design process, and quantify the value AI-driven learning will create.
Innovation can and should be a cycle of continuous refinement and improvement, not a one-shot effort. With machine learning and data science it can, as organizations implement data-driven solutions to enhance key benefits and capabilities.
The possibilities for rapid and effective innovation are practically unlimited. Nearly every device we use on a daily basis from a toothbrush to our favorite belt could potentially leverage data to provide insights to product teams and end users in ways we could never imagine.
For example, when designing the “smart shoe” of the future, a shoe company may think about how sensors contained in the shoe can be used to understand running and walking behavior in order to iterate the next version of the design or provide feedback to users. The data could be leveraged to predict how long the shoes will last, what shoes the wearer may enjoy next, or if product defects are becoming apparent.
It’s important to think through the practical details of embedding advanced data science solutions into product or service development. How will the data will be transmitted, stored, and then leveraged in the context of the product or service ecosystem? For example, will sensor data be transmitted to an app that then uploads it into an enterprise machine learning solution to synthesize, model and score the results to deliver insights? Or will the product be capable of doing the scoring within the device itself? These types of questions require deep understanding of the available data science methodologies and algorithms as well as the design process itself.
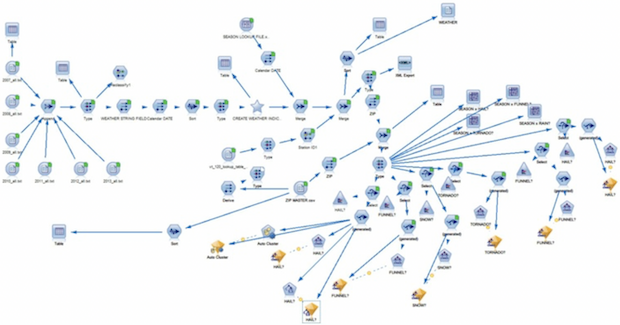
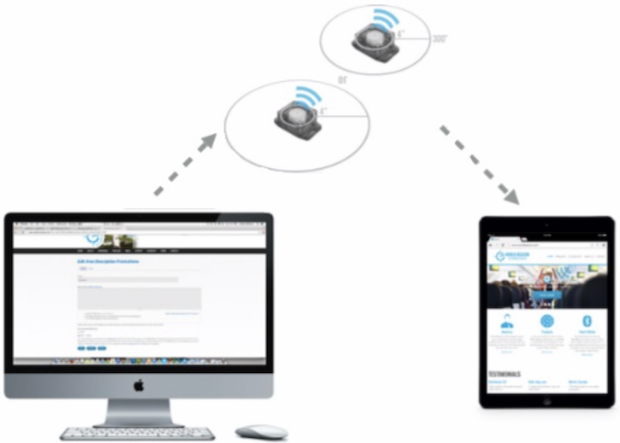
Finally, expand the use of data science to drive operational innovation
Data science and advanced, intelligent analytics can take data from being simply an output displayed on a dashboard or in a standardized report to an integral part of an organization’s planning and execution of new innovations and enhancements to the customer journey.
This largely untapped opportunity resides in how data is leveraged. For example, think of all of the unstructured data in the form of customer comments sitting in spreadsheets and text files throughout your organization. What if that could be used to generate actionable insight in real time? Whether you are collecting customer feedback through a traditional Net Promoter Score survey or looking to leverage operational data that is stored in a traditional business intelligence tool, advanced analytics can help deliver insights and create sustained competitive advantages.
Historically, most companies have tried to generate this insight manually, by having a few employees read through customer comments and try to capture the core thoughts behind the words. While marginally effective for small numbers of comments, this method is timeconsuming, expensive and laborintensive.
While the human brain is still the best tool to quickly understand what a customer is saying and to quantify the varying degrees of satisfaction, we humans have an inherent flaw: we are unable to be truly objective. Each of us processes what we read based on our experiences, feelings, understanding and personal context. Two people reading the same comment can have very different opinions about what it is really trying to say. This method is not very repeatable or even comparable from one day to the next.
Today, algorithms for text analytics allow companies to deliver a scientific and repeatable method of dealing with unstructured content, transforming it into structured data. This use of intelligent automation can enable comments to be analyzed and processed in real time as they are received on social media or customer surveys. The organization gains the ability to react immediately, responding to issues or starting work on potential product improvements.
The new structured data produced from the text analytics can then be used in a predictive analytics model to predict outcomes and customer behavior, with that information leveraged yet again to assess the outcome of changes. Automatically generated insight drives improvements, while making predictions that can be tested to fine-tune the experience.
The bottom line: hindsight gives way to foresight
Data science enables companies to move from relying on hindsight to looking forward. Before data science, this was extremely difficult. Organizations were always looking backward, describing the data in simple terms and generating basic understanding. More advanced interpretation and prediction was an inexact science at best, when it was undertaken at all.
Data science tools and methodologies change all that, by creating the ability to move beyond description to diagnosis, prediction and prescriptive action. The value comes not from simple efficiency, but from foresight… an approach that is truly innovative!
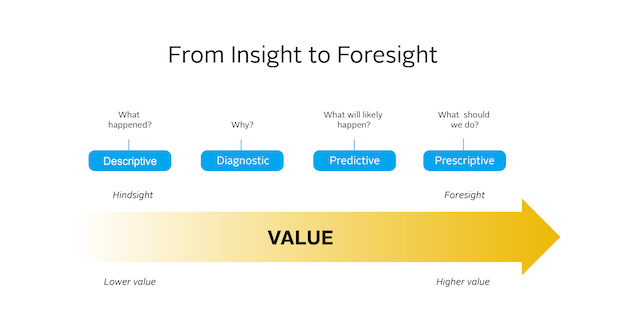
About the author
Kellan Williams is an advanced analytics expert and experienced data scientist with proven analytical acumen. His experience spans many sectors and his skill set has offered him the ability to have high impact roles at IBM Global Business Services, Huntington Bank, Safelite Autoglass, LBrands, and Abercrombie and Fitch.
Kellan has spent the last 12 years helping companies turn their data into actionable insights. Kellan employs advanced methodologies and cutting edge technologies to transform business problems into sustained competitive innovations. He specializes in the areas of text analytics, predictive modeling, and advanced forecasting.